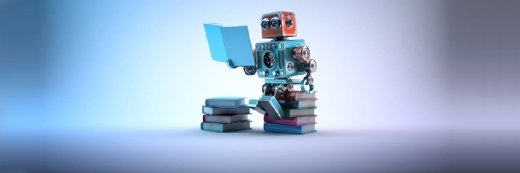
kirill_makarov - stock.adobe.com
The risks of regulating artificial intelligence algorithms
For AI to improve our lives, it needs to reflect the real world, but regulating algorithms to be how we would like them to be risks introducing an unreality that makes them ineffective
The usual people are teaming up with the usual people to try to harness artificial intelligence (AI). That is, Google, Amazon and Microsoft are tying up with the UN, the World Bank and the Red Cross to try to use algorithms to predict famine.
The most important part of this will be getting everyone in such organisations to understand that the point is to measure the world as it is, not as we’d like it to be. This is the basic problem with a certain set of demands about algorithms and AI in general.
Predicting famine is very easy indeed at one level of granularity. As economist Amartya Sen, the great elucidator of the subject to the point that his Nobel prize was largely for this, has pointed out, we have not had a modern famine in a country that is a democracy – actually, with a free press.
People don’t like the idea of either themselves or other countrymen starving, and where that view can be made known through the political process – or the media – then starvation doesn’t happen, and remedial actions are taken.
But, of course, the AI part here is to look at a greater level of that granularity. To feed in information from multiple sources so that we get a heads-up on those things happening that are early warning signs of impending doom. Which is where we’ve got to be the most careful about matters.
Predicting famine
For example, a known predictor of famine is when fresh meat prices are falling rapidly. The cause of this is that farmers note they don't have the fodder to keep herds going, so they send them for slaughter while they can get something for them. The fact that there’s not enough fodder is also a pretty good sign that the next grain crop – and most of the world still gets most of its calories from the local grain crop – is going to be bad to dismal. Thus a surplus of cheap meat is a sign of a food shortage soon enough.
This seems simple enough – feed meat prices into our algorithm. But here is the “be careful” part – we’ve got to feed the free market price in. Feeding the Venezuelan price of any food into our AI isn’t going to tell us anything at all, given that the government there has set all such prices at spit. Which leads, inevitably, to near to no food to be had at any price. Similarly, Soviet prices for foods would not be useful inputs – we would need the black market prices, which reflect availability, to gain anything useful.
In other words, we must use as inputs information about reality, not about the way we’d like the world to be, the manner the law says it ought to be, or even the way nearly all of us say it should be.
It’s this larger point that we have to address. We would all prefer a world without racism: the law says there shouldn’t be any, and yet an AI that assumes it doesn’t exist is not going to be useful. Because, quite obviously, it does exist and any calculation about the world has to acknowledge that. The same is true of sexism, gender discrimination, red lining and any other peril of modern times we want to consider.
Describing the world
We have to describe the world as it is for us to gain useful insights. Sure, we might then use those to convert that reality to how it ought to be, but our ingoing information, plus its processing, has to be morally blind.
There is quite a movement out there to insist that all algorithms, all AIs, must be audited. That there can be no black boxes – we must know the internal logic and information structures of everything. This is so we can audit them to ensure that none of the either conscious or unconscious failings of thought and prejudice that humans are prey to are included in them.
But, as above, this fails on one ground – that we humans are prey to such things. Thus a description of, or calculation about, a world inhabited by humans must at least acknowledge, if not incorporate, such prejudices. Otherwise the results coming out of the system aren’t going to be about this world, are they?
Read more about regulating AI
- Finance firms must keep a close eye on artificial intelligence, according to the Financial Stability Board, an international body that supports financial services regulators.
- Computers can and do make mistakes and AI is only as good its training, so relying purely on machine intelligence to make critical decisions is risky.
- The digital revolution is challenging the regulatory environment across every westernised, developed economy.
Thomas Schelling noted – and his Nobel prize was in part for this – that we only need a very minor preference for racial homogeneity to be able to explain almost entirely racially divided living expanses. If that small preference makes just one household move, that at the margin changes the incentives for the next, which moves, and so on into a cascade. There shouldn’t be such racism and there shouldn’t be such segregation, but it happens – often enough at least – not because everyone is an out-and-out racist.
Just a small and marginal change can be enough to set off the cascade. Should our AI reflect that knowledge – or should it insist on ignoring what we know here?
The insistence also fails in another way. If we actually knew everything that a proper AI was taking into account, then we wouldn’t need the AI, would we? This is the socialist calculation debate all over again.
If we had all the information, and we had it all in real time, with good people processing it the right way, then planned economies would work. But they don’t, so we obviously cannot have all that we need to make them do so.
This is why we use markets – Hayek insisted that the market itself is the only calculating engine, with the correct feedbacks, that can do the working out of the economy for us. Sure, he’s a bit extreme on the point, but again his Nobel was largely for this insight.
Real-world information
We face the same point with algorithms. There are those used for trading these days where no one knows what they’re doing. Sure, it’s possible to look at what they’ve been trading, to tot up profits or losses. But no one really knows what pattern it is the algorithms have spotted that leads to the trades, or what causes such patterns.
They only know that they will trade some set of instruments for a few weeks or months, then stop doing so. The pattern appears to have gone, so they stop. All of which is the very point of using them, of course.
So it will be with larger applications. The very point is that they note and act upon patterns – ones that we can’t see, that we don’t know why are happening. Just as with markets – we might not know why the price of apples has risen, but we’re all getting the information that they have and are acting according to that incentive. The desire to audit all algorithms is missing the very point of having them at all.
AI has to be based on real-world information flowing in. For any result to be useful, the system must operate on the same rules as the real world, imperfections and all, and the very point of our doing all of this is to do what we ourselves cannot.
Any attempts to insist on full audits, moral compliance and preferred data aren’t just going to fail – they’re missing the entire point of the exercise.