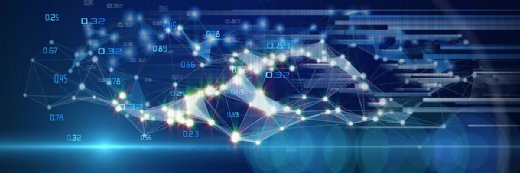
Anatoly Stojko - stock.adobe.com
Building better data foundations to make the most of AI
The UK government's national artificial intelligence strategy relies on businesses putting in place the foundations for better use of data – EY research highlights the challenges ahead
In September 2021, the government published its inaugural National AI Strategy, an ambitious 10-year vision for the UK’s digital future. As well as the requirement to improve governance and regulation of artificial intelligence (AI), the strategy recognises that increasing resilience, productivity and economic growth will require businesses across the UK to use data much more effectively than they are now.
To achieve this, companies in every region and sector need to ensure their organisation’s data is fit for purpose, recorded in standardised formats on modern, future-proof systems and stored in a way that makes it findable, accessible, interoperable and reusable. If these basic data foundations are not constructed properly, then efforts made to build new AI services and products will inevitably be undermined. Ultimately, poor data foundations will compromise trust in data and may mean that the UK fails to deliver on its AI vision.
What are the barriers holding back businesses from building better data foundations, and how can these be overcome? To answer these questions, EY was appointed by the Department for Digital, Culture, Media and Sport (DCMS) to gather evidence to assess the extent of data foundations and the adoption of AI in the UK.
The research was based on surveys, interviews and a rapid review of other high-quality reports and evidence. Businesses were asked about the extent to which data foundations and AI were being adopted and the barriers to their use. To ensure that the research was as representative as possible, it included points of view from across the UK economy – from large businesses, to small and medium-sized enterprises (SMEs), and organisations in the third sector.
Although our survey showed that 80% of organisations believed that improving data foundations would increase productivity, there is still a sizeable gap between the potential and actual impact of data to drive long-term economic value.
We concluded that, despite accessibility, interoperability and usability of data generally being regarded by organisations as good, it was clear that many data-related initiatives have yet to reach full maturity. Indeed, businesses of all sizes and from all sectors believed they could be more successful if improvements were made in the following areas:
Quality of data – 41% of organisations in the survey selected quality as the most challenging characteristic of their data, and 90% said they had a dedicated data strategy or data-improvement initiatives in place.
Skills – 14% of organisations said that recruiting and retaining appropriately skilled personnel was their biggest current challenge, and 79% said the government’s main priority should be investment in developing relevant data skills.
Infrastructure – 14% of organisations cited challenges with access to suitable technology and data infrastructure, and fixing issues with legacy IT systems.
Read more about AI and data
- Big Data London: Mitigate AI bias rather than try to remove it, say experts.
- Emerging trend expands data science capabilities to more people using AI.
- ICO launches guidance on AI and data protection.
EY’s research also highlighted a divide between large businesses and SMEs (and the third sector). SMEs are more likely to be profoundly impacted by issues linked to data foundations. For example, they tend to struggle more than large companies to access suitable technology and data infrastructure, and they can’t always compete in what is already a difficult jobs market for data skills.
This means that SMEs tend to lag behind larger companies in their adoption of AI. This is borne out in the results of our survey: although 90% of large organisations have already adopted AI or plan to do so soon, this drops to less than half (48%) for SMEs.
By drawing on a mix of technological and cultural approaches, businesses across all regions and sectors, and of all sizes, can improve their ability to use their data more effectively. For example, businesses can:
Build trust in data: By adopting robust data governance processes coupled with a data fabric – a set of independent software services from the field of AI that create a consistent and expanded data experience across the enterprise – businesses can track data throughout its life. This, in turn, ensures its secure ingestion, processing and use, reducing excessive movement, copying and inconsistent use, eliminating duplication and implementing data quality and control protocols to ensure that all data and AI they support can be trusted.
Tap into new pools of talent: By activating the apprenticeship levy, businesses can access young talent and shape the digital skills needed for them to remain competitive. Also, upskilling and reskilling existing employees helps introduce new skills into an organisation and enables people to shift from routine activities to value-adding work. Businesses can also build and maintain more flexible access to skills through contractors and freelancers.
Switch to “platform thinking”: By adopting a cloud-based approach, particularly when combined with a data fabric, businesses can simplify application integration so that data can move around an organisation to be enriched, processed and visualised at any point of need rather than remaining locked up in legacy silos or being replicated across multiple applications.
It is difficult to predict just how much value can be unlocked in the economy by having better data foundations to increase the adoption and effectiveness of AI. But EY’s research suggests that businesses do understand the correlation between better use of data and the increased economic and social value that can be realised using AI. The reality, though, is that they have little choice – no other resource, natural or artificial, provides the same degree of potential as data.
Harvey Lewis is associate partner and chief data scientist in EY’s tax practice