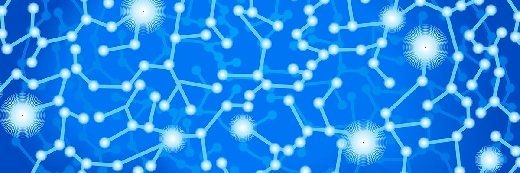
Fotolia
Google vows to simplify creation of custom machine learning models for developers
Google’s ongoing push to lower the barriers to machine learning and artificial intelligence adoption for enterprises moves up a gear with custom model creation
Google has set its sights on speeding up the time it takes enterprises to create custom machine learning models through the imminent launch of its Cloud AutoML service suite.
The services are designed to build on the momentum the search giant claims to have seen to date with its push into the machine learning space.
This has previously seen the firm debut a mix of application programming interfaces (APIs), dubbed Vision, Speech, NLP, Translation and Dialogflow, that developers can combine with pre-trained machine learning models to advance the functionality of their business applications.
According to Fei-Fei Li, chief scientist for Cloud AI at Google, these pre-trained machine learning models have limited the ability of some businesses to tackle industry-specific problems using artificial intelligence (AI).
“We’ve made it easier for [businesses] to implement machine learning models by offering AI building blocks for text, speech, natural language, video and vision via our machine learning APIs,” she said at the Cloud AutoML press conference.
“To ensure these solutions work in a range of situations, these models needed to be pre-trained. Businesses can then use these as the basis for their solutions, but the reality is – for businesses in retail, healthcare and finance, [for example] – that customers still need to customise their models.
“These building blocks only offer generic solutions. It doesn’t solve the specific problems for different industries in different scenarios,” she added.
In light of this realisation, the company is now bringing to market a set of tools designed to lower the skills barrier for developers who want to create applications based on more complex and custom-made machine learning models.
Cloud AutoML Vision is the first of these tools Google is planning to bring to market, and is geared towards speeding up the time it takes developers to create custom machine learning models for image recognition tasks.
The company claims the setup has been shown to make fewer mistakes when identifying images of objects uploaded to the service than those based on more generic machine learning APIs.
Moving on with machine learning
The announcement marks the latest step in the search giant’s ongoing quest to democratise AI and machine learning technologies, so more enterprises can tap into the benefits of using them.
Read more about Google and its machine learning strategy
- Fei-Fei Li, Google's chief scientist of artificial intelligence and machine learning, talks up societal benefits of making artificial intelligence tools and technologies accessible to all.
- Ocado’s head of data, Dan Nelson, reveals details of how the online grocer is using machine learning to bolster the responsiveness of its customer service teams.
While the company has made some progress on this front, Li said the skills that businesses need to really take advantage of AI and machine learning are still in short supply.
“AI and machine learning is still a field with high barriers to entry, and it requires rare expertise and resources that few companies can afford on their own,” she added. “Only a handful of businesses in the world have access to the talent and budgets to fully appreciate the advancement of machine learning and AI.”
There are only thousands of deep learning researchers today, and perhaps a million data scientists worldwide. However, there are an estimated 21 million developers today, and we want to make AI accessible to all of these developers,” she added.
Conservation in the cloud
A follow-up blog post to the announcement shared details of how some of Google’s customers, including Zoological Society of London (ZSL), are already making use of Cloud AutoML.
Sophie Maxwell, the conservation charity’s technology lead, said Cloud AutoML is assisting with the society’s research into the geographical spread of wildlife populations around the world, and how these are being affected by external factors, such as human-related impacts.
“ZSL has deployed a series of camera traps in the wild that take pictures of passing animals when triggered by heat or motion. The millions of images captured by these devices are then manually analysed and annotated with the relevant species, such as elephants, lions and giraffes, which is a labour-intensive and expensive process,” she said.
“ZSL’s dedicated Conservation Technology Unit has been collaborating closely with Google’s CloudML team to help shape the development of this exciting technology, which ZSL aims to use to automate the tagging of these images – cutting costs, enabling wider-scale deployments and gaining a deeper understanding of how to conserve the world’s wildlife effectively.”