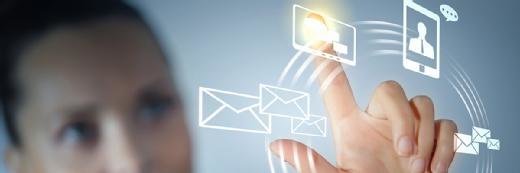
Sergey Nivens - Fotolia
Dell CTO: Enterprise AI poised to take off in 2025
After a period of experimentation, the building blocks are in place for wider enterprise adoption of AI, says Dell Technologies’ global CTO, John Roese
The enterprise artificial intelligence (AI) market is poised to grow next year, driven by the maturation of key technologies and availability of off-the-shelf tools, according to Dell Technologies’ global chief technology officer and chief AI officer, John Roese.
In an interview with Computer Weekly in Singapore, Roese painted an AI landscape that comprises three distinct, yet interconnected markets: pre-generative AI (GenAI), AI model training and enterprise AI.
While the first two markets are well established, it’s the enterprise AI market that holds the greatest potential, albeit with a slower adoption curve, he noted.
“AI is not a single market – it’s three completely independent markets that are related,” Roese explained, noting that pre-GenAI technologies like computer vision and robotics act as a crucial layer that distils data into formats usable by GenAI.
The second market, focused on training massive AI models, is currently dominated by hyperscalers, as well as tech giants vying for supremacy in search and social networking, with Dell playing a key role in supplying the necessary infrastructure.
However, it’s the third market – enterprise AI – that Roese believes is on the cusp of transformation, noting that while the training market grabs headlines with massive investments in “mega clusters” by tech giants, the enterprise market is more focused on applying AI to business processes.
“Enterprise AI is about understanding which processes in your enterprise make your enterprise work, and with the application of AI, being able to do those processes materially better,” he said. That means using AI to optimise supply chains, improve customer service and enhance product development.
One key difference, according to Roese, is that enterprises are primarily focused on inference – running data through existing models – rather than training their own models. This will require a different infrastructure approach: one that’s distributed, optimised for inference and potentially extending to the edge.
“We’ve seen a maturing of the enterprise market in the last six months,” Roese observed, pointing to the rise of off-the-shelf AI tools and capabilities aimed at enterprises, which are generally better at consuming than producing technology.
Six core capabilities
Roese outlined six core capabilities that can address the majority of enterprise AI use cases, urging chief information officers to focus on these foundational elements rather than build a swathe of capabilities. This will help them to prioritise AI investments in areas that directly leverage their core competencies and competitive advantage.
The six capabilities include retrieval augmented generation (RAG)-based chatbots, coding agents, content AI engines, data management agents, analytics agents and fine-tuning infrastructure. Roese said these capabilities were sufficient to implement over 300 AI use cases at Dell today.
Specifically, the rise of agentic AI architectures represents a significant turning point. These architectures comprise smaller, specialised AI models that act like a “digital workforce” to perform specific tasks, offering greater control, explainability and the ability to incorporate proprietary data through fine-tuning.
“You’re effectively building the equivalent of teams of people with different skills,” said Roese. This allows for a more collaborative approach between humans and AI, where humans orchestrate and oversee the work of these digital agents.
Roese expects agentic architectures to become more mainstream with more standardisation, driving enterprises to adopt agent-oriented AI systems from the middle of 2025. “That will be probably the first time we start to see significant acceleration in the production of AI in the enterprise,” he said.
Addressing concerns around data leakage and agent management, Roese said different types of agents will require different lifecycle management approaches.
For example, agents with stable, specific tasks can be managed in the same way as updating packaged software, while those that interact with dynamic, real-time data should use RAG to access the data, rather than embedding the data in the agent itself.
Organisations can also use an ensemble of specialised agents, rather than a single monolithic agent, for better control and explainability. Each agent in the ensemble can have a different management lifecycle tailored to its specific function.
For instance, a communication agent running on a local machine can use tools like LangChain to talk to a monolithic large language model or an agent that can access real-time information about an organisation’s inventory. “It’s the collaboration between the two agents that gives you what appears to be a real-time answer, even though from a lifecycle perspective, the only thing that’s real-time is the database behind the second agent,” said Roese.
He acknowledged the challenges enterprises face in navigating the complex AI landscape, citing governance, the influence of “random vendors” over business units that are driving most AI projects, and the need for predictable costs as key hurdles.
Still, Roese remains optimistic about the future, advising companies to focus on strategic AI implementations that align with their core competencies and leverage readily available off-the-shelf tools. “As you put better governance in place, as things become more off-the-shelf, you can actually move faster,” he said.
Read more about AI in APAC
- Some 500 customer service officers at Singapore’s DBS Bank will soon be able to tap a GenAI-powered virtual assistant to improve workflows and better serve customers.
- Snowflake’s regional leader Sanjay Deshmukh outlines how the company is helping customers to tackle the security, skills and cost challenges of AI implementations.
- The Australian government is experimenting with AI use cases in a safe environment while it figures out ways to harness the technology to benefit citizens and businesses.
- Pure Storage’s global CTO discusses the data and sustainability challenges in AI adoption, which can be addressed by centralising datasets and focusing on data quality and management.