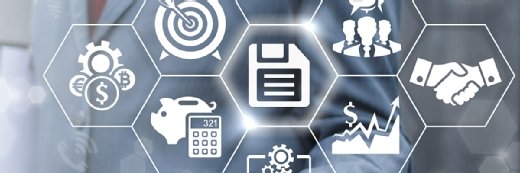
wladimir1804 - stock.adobe.com
How Pure Storage is supporting AI workloads
Pure Storage’s cloud-compatible and energy-efficient modular flash storage architecture is well-poised to address the data storage demands of artificial intelligence workloads
The increasing use of artificial intelligence (AI) is driving more enterprises to re-evaluate their storage architectures to keep pace with the demanding nature of AI workloads, which typically have higher computing and data storage requirements than generic tasks.
One notable challenge is the substantial volume of data needed for training and refining AI models. This necessitates storage systems that are not only scalable, but are also consistently accessible with lower latency and higher throughput to enhance workload efficiency.
Many storage providers in the market have been addressing the demands of AI workloads for a considerable time. For Pure Storage, a company committed to advancing the transition towards all-flash datacentres, these efforts encompass both hardware and software.
Speaking to Computer Weekly on a recent visit to Singapore, Amy Fowler, vice-president and general manager of FlashBlade at Pure Storage, noted that many AI applications now run in containers, which means those workloads need to be cloud-compatible, allowing one to spin up or spin down a Kubernetes environment.
“Portworx is very well-suited to that, and we have very large customers, including household-name retailers, that are using Portworx for those types of projects,” she said. Portworx, a Kubernetes data services platform, was acquired by Pure Storage in 2020.
On the data front, Fowler said Pure’s recently launched FlashArray//E, which offers flash-like performance at the cost of hard disk drives, is suited for structured data stored on block and file storage, while the FlashBlade family enables storage to be disaggregated from compute.
“Instead of integrating storage with the GPU [graphics processing unit], the storage is external and shared by many different GPUs,” Fowler said, noting that this approach is more efficient as it utilises a shared data source, eliminating the need to transfer data between different systems.
“You’ll have a smaller amount of equipment and when the storage is very high performant in a machine learning environment, GPUs can operate at their maximum capacity rather than being underutilised,” she explained.
With AI workloads generating substantial heat, which leads hyperscalers such as Microsoft to consume more water primarily to cool its datacentres, energy efficiency is a vital consideration for enterprises that run AI workloads.
Fowler said Pure has been optimising its storage products for efficiency, beginning with its DirectFlash module that enables all-flash arrays to communicate directly with raw flash storage instead of using commodity solid-state drives (SSDs).
Apart from using less material to build FlashBlade and FlashArray systems, compared with other SSD-based all-flash systems (and much fewer materials than disk-based systems), Pure has been prioritising both data deduplication and compression to maximise effective raw storage capacity.
“We then put these into the smallest form factors that require the least amount of power, which means they need the least amount of water to cool the systems,” said Fowler.
The modularity of Pure’s flash storage architecture also lets enterprises buy and consume what they need to run their AI workloads, which can be unpredictable.
“They can upgrade components without throwing out the whole system. So, if they start with an X50 because that’s the amount of performance and capacity they need, but later realise they need more performance, they can non-disruptively trade in their controllers and upgrade to an X70 or X90,” said Fowler.
Pure’s Evergreen//One storage-as-a-service platform can further enhance cost-effectiveness by offering a consumption-based model, Fowler noted: “We know AI is not going away, but no one knows exactly what and how many models they need to build. So, it makes a big difference on the cost to run these systems.”
South Korean messaging platform Kakao has been using Pure’s FlashBlade storage systems to support research teams that are building AI models for its services. Il-seop Jin, manager of Kakao’s infrastructure planning team, said the company has been able to eliminate storage bottlenecks, use data more efficiently and lower the cost of managing infrastructure.
Read more about storage in APAC
- In this expert guide to storage management in Asia-Pacific, we unpack the developments shaping storage management and key strategies to extract business value from data.
- Lenovo’s head of storage in Asia-Pacific singles out the region’s market opportunities and predicts that the healthcare sector will go full-fledged with flash storage in the next year.
- NVMe storage is becoming popular among Asia-Pacific enterprises that want to reduce latency and speed up application performance.
- Liverpool Football Club has transitioned from on-premise infrastructure to Wasabi cloud storage that’s well-suited to its video production and distribution requirements.