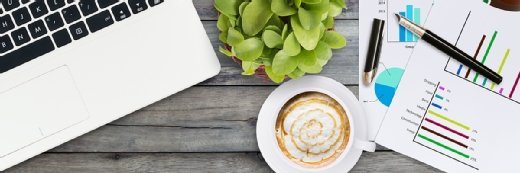
blackzheep - stock.adobe.com
Getting data analytics right
Organisations will need to marry the art and science of data analytics to drive innovation in their analytics initiatives, according to Gartner
From understanding consumer behaviour to optimising parcel delivery routes, data analytics applications have become embedded in everyday life for many people and businesses.
But underpinning the capabilities of such applications aren’t always the most advanced artificial intelligence (AI) algorithms, said Peter Krensky, director and analyst at Gartner’s business analytics and data science team.
For one, organisations can drive good business outcomes with augmented analytics, which Gartner defines as the use of enabling technologies such as machine learning and AI to assist with data preparation, insight generation and insight explanation to augment how people explore and analyse data in analytics platforms.
Augmented analytics also augments experts and citizen data scientists by automating many aspects of data science, machine learning, and AI model development, management and deployment.
Speaking at the Gartner Data and Analytics Summit in Sydney, Australia, Krensky noted that while 82% of organisations have adopted augmented analytics or plan to do so by the end of 2022, not enough organisations are using these new capabilities to their full advantage.
“Too often, we’re using these new tools to do old tasks like running reports and creating charts,” Krensky said, calling for organisations to use augmented analytics to uncover patterns and outliers in their data that merit further investigation.
“Analytics is a science in which we continually improve our understanding and develop new techniques to refine it even further,” he added. “AI already enables all kinds of life-enhancing use cases, like smart farming and agile supply chain optimisation.”
However, the power of analytics can also create false expectations and put unnecessary burdens on analytics teams, especially for those that seek to maximise their use of analytics to find the best answer to their questions.
“They look at all the options, check all the data and run all the numbers and that does absolutely give the best answer,” said Sally Parker, research director in Gartner’s chief data officer leadership team.
“But the truth is that even a maximising strategy does not yield the perfect answer, just the best one that we might be able to identify with the data we have, which is almost always incomplete or imperfect,” she added.
Against this backdrop, Krensky said a “satisficing” strategy might be more feasible in finding a satisfactory or sufficient answer with the data one has, an approach that is not heard often enough.
Analytics as an art
The science of analytics is well understood, but organisations should also see analytics as an art “because art is a lens for interpreting the world in different and often insightful ways”, said Parker.
Parker said that requires analytics teams to identify the right questions, and not simply the right answer, because one purpose of art is to provoke and challenge, which is generally achieved better with questions than answers.
“Good analysis, like good teachers, answers questions, but just like great teachers, great analysis can prompt us to ask new and better questions,” she added.
For example, Parker said while “Who is buying a product?” is a question that can be easily answered with a maximising strategy, a great question would be: “Who is buying our product that looks nothing like the customers we know and understand?”, because the answer would help an organisation identify new market segments.
“Data artists create great questions, just like data scientists create great answers, and we need both,” she added.
That said, analytics practitioners will also need to know when and if an answer is good enough.
“For a decision-maker, an approximation in the next five minutes could be more valuable than the exact right answer in five hours,” Krensky said. “And remember, every question answered potentially leaves a different question unanswered, so we have to constantly ask if we want to spend another hour answering this question or spend that hour investigating a different question.”
In some cases, organisations might not even find an answer to their questions. Take, for example, the Brussels Intercommunal Transport Company, which had hypothesised that its buses were breaking down unpredictably due to the bad driving techniques of its drivers.
Krensky said the company’s business intelligence (BI) team sought to solve the problem and applied the Scrum technique of time-boxing, spending no more than 10 days on data preparation and no more than 10 days on model development.
The plan was to use the buses’ telemetry data to predict breakdowns by applying deep learning techniques, but the team could not find any pattern at all.
“Because they had time-boxed their project and accepted the risk of failure associated with this kind of innovation, they were able to move on instead of getting bogged down by trying to prove a point.”
Krensky said the good thing that came out of the project was that the BI team had built a trusted relationship with their operations colleagues who could see that they had the integrity to not force a result when they couldn’t find one.
In addition, the BI team had identified a list of buses that run in the hilliest parts of Brussels, and by rotating those buses to run in every part of the city, the company was able to save €40m a year.
Read more about data analytics in APAC
- More data is not always better, says Gartner, which is calling for organisations to focus on metadata and synthetic data to reduce their data liability and address privacy challenges.
- Globe Telecom, the largest telco in the Philippines has moved its on-premise data warehouse to Snowflake to address scalability challenges and drive its data analytics initiatives.
- Banks and telcos contributed nearly one-third of spending on big data analytics in APAC, while the government and healthcare sectors will register the highest growth rates.
- Global financial institution Citi puts people at the centre of its data analytics initiatives and collaborates with users to drive adoption and success.