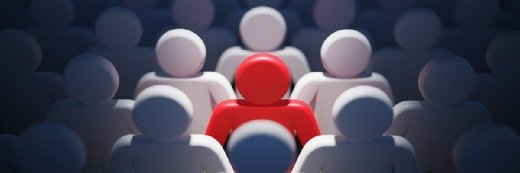
vchalup - stock.adobe.com
Twitter investigates image cropping algorithm for racial bias
The algorithm’s consistent favouring of white faces in image previews has forced the company to investigate it for racial bias
Twitter is investigating racial bias in its image cropping algorithm, after users discovered it prioritises white people’s faces over black people’s faces.
Users first noticed the issue when Vancouver-based university manager Colin Madland posted on the social media platform about his black colleague’s trouble with Zoom’s face-detection algorithm, which kept removing his head whenever he used a virtual background.
When tweeting pictures of the Zoom interaction, Madden found the Twitter mobile app consistently defaulted to his face instead of his colleague’s in the preview. This occurred even when he flipped the order of the images.
The discovery prompted a slew of experiments by other Twitter users, which ended with similar results.
For example, when white US senate majority leader Mitch McConnell’s face was placed in an image with black former US president Barack Obama, it was the former’s face that was prioritised for the preview.
Other users did the same experiment with fictional cartoon characters Lenny and Carl from The Simpsons, ending in the same result.
However, according to Twitter, the algorithm had already been tested for both racial and gender bias before going live and found no evidence of either. “But it’s clear that we’ve got more analysis to do,” it said. “We’ll continue to share what we learn, what actions we take, and will open source it so others can review and replicate.”
Public test
Twitter’s chief technology officer, Parag Agrawal, added: “We did analysis on our model when we shipped it, but it needs continuous improvement. Love this public, open and rigorous test – and eager to learn from this.”
In a since-deleted thread, Twitter user and German developer Bianca Kastl posited that the algorithm could be cropping the images based on “saliency”, which is described in a January 2018 blog post from Twitter as a region of a picture “that a person is likely to look at it when freely viewing the image”.
“Academics have studied and measured saliency by using eye trackers, which record the pixels people fixated with their eyes. In general, people tend to pay more attention to faces, text, animals, but also other objects and regions of high contrast,” said Twitter in the post.
“This data can be used to train neural networks and other algorithms to predict what people might want to look at. The basic idea is to use these predictions to centre a crop around the most interesting region.”
Twitter’s chief design officer, Dantley Davis, said “contrast can be problematic” for the algorithm in certain situations, and that the company is reassessing its model based on the feedback it has been getting from users.
“I’m as irritated about this as everyone else. However, I’m in a position to fix it and I will,” he tweeted, adding separately: “It’s 100% our fault. No one should say otherwise. Now the next step is fixing it.”
Read more about technology and ethics
- Strict ethical and professional standards should be applied to the development of algorithms with social impacts to recover public trust in the technology, according to a report by BCS, the Chartered Institute for IT.
- The Home Secretary has pledged to stop using an algorithmic decision-making system to assess visa applications after migrant welfare and technology justice groups challenged the tool for helping to create a hostile environment for migrants.
- In response to the sudden and widespread adoption of masks, facial-recognition companies across the globe have been busy developing a range of new algorithms that can identify people with “occluded” faces.
According to Gemma Galdon Clavell, director of Barcelona-based algorithmic auditing consultancy Eticas, the case of Twitter’s image-cropping algorithm identifies a number of major issues her firm views as critical when auditing algorithms.
The first of these is that simply testing for bias alone is not enough – the results should be published as part of an audit, as “only then can users assess whether the efforts made are enough to ensure algorithms are mitigating bias”.
She added that algorithms are also often tested in lab environments, the results of which developers assume will be replicated in real-world contexts. As such, she told Computer Weekly that “bias auditing should be a continuous exercise”.
“When using machine learning, ‘testing’ at the beginning is also not enough. As the algorithm learns, the biases of real life dynamics and technological shortfalls of algorithmic models end up being replicated by the system,” she said. “It is particularly concerning that Twitter reps have struggled to explain how the algorithm learns bias, and this points to a fundamental problem: how to protect people in systems not even their creators understand or can hold accountable?
“While the efforts made by Twitter to at least identify bias are commendable, it is increasingly clear that automation raises serious concerns that deserve a lot more attention, resources and specific methodologies that shed light on the black box of algorithmic processes.”
For Charles Radclyffe, an AI governance specialist and partner of environmental, social and corporate governance (ESG) benchmarking agency Ethical by Design, the pertinent question is why these mistakes always seem to prejudice people of colour.
“The technology industry is structurally racist, and we need to urgently change it,” he said. “Data sets are biased, developer teams are mostly un-diverse, and stakeholders such as customers tend to have nowhere to raise their issues and be heard. Finding technical workarounds to these issues is not going to solve the problem. AI ethics needs to be seen as a corporate governance issue, and measured and managed in the same way.”