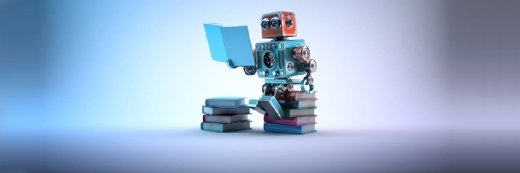
kirill_makarov - stock.adobe.com
Build 2020: How Microsoft aims to build trust in artificial intelligence
Microsoft has outlined its toolset for making AI models explainable with the InterpretML and AboutML tools
During its annual Build 2020 developer conference, Microsoft outlined plans for responsible development processes for machine learning. The aim is to make these repeatable, reliable and accountable.
Azure Machine Learning automatically tracks the lineage of datasets, so customers can maintain an audit trail of their machine learning assets, including history, training and model explanations, in a central registry. This gives data scientists, machine learning engineers and developers improved visibility and auditability in their workflows.
During the conference, Microsoft provided guidance on how custom tags in Azure Machine Learning could be used to implement datasheets for machine learning models, enabling customers to improve the documentation and metadata of their datasets. Custom tags are based on Microsoft’s work with the Partnership on AI and its Annotation and Benchmarking on Understanding and Transparency of Machine Learning Lifecycles (About ML) project, which aims to increase transparency and accountability in the documentation of machine learning systems.
Another area of focus for Microsoft is the tools that build a better understanding of machine learning models and assess and mitigate unfairness in data. It has been investing heavily in tools for model unfairness and explainability over the past few months. These areas are of particular importance to practitioners of machine learning at the moment.
Read more about AI tools
- Microsoft AI Builder empowers non-programmers to incorporate AI as they build applications using low-code or no-code platforms.
- An OpenAI partnership with Microsoft aims to provide OpenAI with an influx of capital to boost its AGI research and give Microsoft new supercomputing Azure AI capabilities.
In 2019, Microsoft released Fairlearn, an open source toolkit that assesses the fairness of machine learning models. At this year’s Build, Microsoft announced that it would integrate the toolkit natively into Azure Machine Learning in June.
The Fairlearn toolkit offers up to 15 “fairness metrics” against which to assess and retrain models. It also offers visual dashboards to show practitioners how the model is performing against certain groups selected by the customer, such as age, gender or race. Microsoft plans to add to these capabilities as research in the field progresses.
It is also addressing explainable artificial intelligence (AI) with a tool called InterpretML, which offers a set of interactive dashboards that use various techniques to deliver model explainability. For different types of model, InterpretML helps practitioners better understand the most important features in determining the model’s output, perform “what if” analyses and explore trends in the data.
Microsoft also announced that it was adding a new user interface equipped with a set of visualisations for interpretability, support for text-based classification and counter-factual example analysis to the toolset.
Visualising explainability for customers
The emerging nature of these crucial areas in AI has made it challenging for some early-stage enterprises to understand how the technologies work in practice, especially for their customers.
Microsoft has made a host of visual improvements in this area, particularly for data scientists and machine learning engineers. In a demonstration of InterpretML, Microsoft showed how a retailer could use explainability in action, for example on its website to support its AI-driven product recommendations for consumers. Building transparency and trust in AI among customers is one of the biggest hurdles for the technology at the moment.
In fact, trust – or a lack of it – in the technology has emerged as the biggest barrier to adoption of machine learning in enterprises. In a CCS Insight survey in 2019, 39% of IT decision-makers cited trust as the biggest hurdle to adoption in their organisation.
Nicholas McQuire is a senior vice-president and head of enterprise and AI research at CCS Insight.