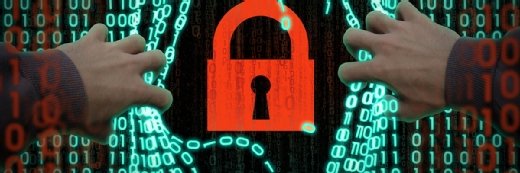
valerybrozhinsky - stock.adobe.c
Microsoft talks up benefits and pitfalls of machine learning in security
Software giant Microsoft uses machine learning models to detect emerging threats while keeping an eye on potential bias in security data points that could derail its analysis
Making sense of trillions of security signals collected by Security operations centres can be a gargantuan task for security analysts if not for machines that help to flag up malicious activities before they turn into full-blown cyber attacks.
At Microsoft, for instance, 6.5 trillion security signals pass through its Azure cloud service every day, and while the software giant employs hundreds of security professionals, parsing that many data points is beyond what humans can do, said its cyber security field CTO Diana Kelley.
“That’s where artificial intelligence and machine learning [ML] can come in to help us find early information about attacks and see things that humans can’t see,” Kelley told Computer Weekly on the sidelines of RSA Conference Asia-Pacific and Japan held in Singapore last week.
Microsoft uses techniques like compound detection and Monte Carlo simulations to turn low fidelity signals into high fidelity ones. And as the machine learns, security events that may not be apparent to people can be picked up over time.
Kelley said in some cases, threats can be detected in milliseconds, while at other times it could take minutes in the case of the Bad Rabbit ransomware that drew comparisons with WannaCry and NoPetya which cost some victims millions of dollars in lost revenue.
Microsoft encountered Bad Rabbit on a Windows device and put it through multiple ML models on the Azure cloud.
It took 14 minutes to establish that the malware was truly malicious – a response that Kelley noted was “very rapid when you think about a human actually going in and trying to do the reverse engineering on it”.
Although ML helps to speed up the threat response cycle, without diverse teams working on ML models, the data and threat models may end up automating bias or even the attack paths for criminals.
“You have to make sure that you've got cognitive diversity,” Kelley said. “The teams that are building these models should not only include data security scientists and computer engineers, but also people like lawyers and privacy experts so you can take a holistic approach in creating ML models.”
On the data side, Kelley warned about unconscious bias that could result in biased data being used to train ML models.
Using an example of a ML tool that automatically parses CVs to identify the top candidates, Kelley said there would already be bias in the data if an organisation hires mostly male employees.
“In computer engineering and cyber security where it’s predominantly male, the tool would think that males are better candidates because they are hired much more frequently,” Kelley said.
“This is also seen online with financial information shown to users – when a user is a woman, she is less likely to get information about new investment opportunities because traditionally, men have done more investments.”
Cyber attackers are already starting to manipulate data used by ML models to throw their victims off-course.
“They’ve tried some pretty interesting attacks, like changing just a pixel on a picture that’s going to be classified, because that can change the classification to a human,” Kelley said. “They are trying to understand how the classification occurs so that they can feed it.”
According to Gartner, early uses of artificial intelligence and ML in security have been in areas such as malware classification, user and entity behaviour analysis, as well as endpoint security. The technology research firm expects machine learning to become a normal part of security strategies by 2025.
Read more about cyber security in APAC
- The onslaught of cyber attacks being reported each day has been a wake-up call, but APAC experts say businesses need to be mindful of the limitations of certain security measures.
- Even as Southeast Asia works towards coordinating cyber security strategies, more needs to be done to establish cyber norms.
- Healthcare organisations in the Asia-Pacific region could lose an average of $23.3m to cyber attacks, including losses from productivity and customer churn, a study finds.
- Australia’s privacy watchdog recorded over 800 cases of data breaches, nearly one year into the country’s mandatory data breach notification regime.