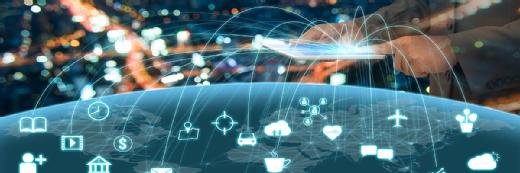
kwanchaift - stock.adobe.com
Davos 2019: Why data sharing is key to AI in Industry 4.0
Machines can learn from humans, but no one does the same job in the same way, so it makes sense to identify best practices
Artificial intelligence (AI) is set to power the next stage of Industry 4.0, as manufacturers develop smart factories, digital twins of physical assets and deploy machine learning.
Analyst Gartner has forecast that AI growth is accelerating. “Four years ago, AI implementation was rare – only 10% of survey respondents reported that their organisations had deployed AI or would do so shortly,” said Chris Howard, distinguished research vice-president at Gartner. “For 2019, that number has leapt to 37% – a 270% increase in four years.
“If you are a CIO and your organisation doesn’t use AI, the chances are high that your competitors do and this should be a concern.”
Among the hot topics discussed during the World Economic Forum (WEF) in Davos is the use of AI and, in particular, industrial AI, to drive reliability and empower new business models.
In an article published at the start of the WEF, Roland Busch, CTO at Siemens, wrote: “The keys to success in the digital age are speed and scale. And if there’s one area in which AI is already far ahead of us humans, it’s the tremendous speed at which models process data and then detect and exclude errors. In short, AI has the potential to help us avoid mistakes and overcome coincidence.”
Siemens has been a pioneer in Industry 4.0, where automation is being used to run smart factories. Busch regards AI, through industrial AI, as the next phase in the reinvention of traditional manufacturing.
“With Industry 4.0, we have successfully started the digital transformation,” he said in the article. “With industrial AI, we can now take it to a whole new level. We can outpace error and coincidence. We can drive innovation. We can increase efficiency and productivity. We can shape technological and social progress.”
Challenges of supervised machine learning
The complex process in aircraft assembly, where torque wrenches and rivet tools need to be used at specific settings in a highly controlled manner to attach panels to an aircraft such as the Airbus A320, could be considered a good candidate for machine learning.
But Sébastien Boria, mechatronics and robotics technology leader at Airbus, said: “To have efficient machine learning, you need supervised systems for collecting huge amounts of data.”
Read more about Industry 4.0
- Creating a smart factory needn’t require starting from scratch. Here is advice on how to create connectivity with the industrial machines you already have.
- Making legacy, often proprietary, systems smart is not as easy as 1, 2, 3. Interoperability and teamwork are key to making Industry 4.0 a reality, says oneM2M’s Chris Meering.
This is most apparent where machine learning systems are used to observe how human operators perform a particular manual function, in order to learn how to automate the job, or work out how it can be performed more efficiently.
For Boria, the challenge is that the AI algorithm can only learn how human operators work. “The main problem with supervised machine learning is that at some point, if you base the results on someone who is not the top performer, you end up with results that are average,” he said.
An AI system that takes a sample of how all people who work on in this particular manual process do the same job, may well come up with an average answer, but for Boria, average does not necessarily translate into the best way to achieve the same result.
“Technology is not a democracy,” he said. “Just because 50% of the population does something, does not make it the right solution.” Worse still, he said, the top performer’s results “may be seen by the machine as an anomaly”.
At a more granular level, every data point or parameter that needs measuring requires a sensor. “You need to connect the physical world with the digital world,” said Boria.“Without data you cannot do anything. This means you need sensors and electronics to capture data.”
But according to Boria, it is simply not possible to manage all the possible parameters that can be collected during aircraft construction. Some types of sensor are too expensive or do not work well when deployed wide-scale.
Wearables not cost-effective
For instance, the collection of some data, such as using smart glasses to see the work being done, are simply impractical, said Boria. “Wearables are not cost-effective gadgets,” he said. “We are looking for products that will give the right user experience. Having looked at all the glasses on the market, I think people don’t want to wear these systems on their eyes for an eight-hour shift.”
Chen Linchevski, CEO and co-founder of Israeli AI startup Precognize, took part in a WEF panel discussion looking at the impact of AI. He said: “To remain competitive, companies need to take a predictive approach to quality, improving operations and efficiency and environmental constraints. Maximising investment in AI- and human intelligence-based technologies is critical. In the long run, it is less expensive to deploy software that anticipates and handles problems before they shut down production.”
Precognize combines human knowledge and machine learning to create what Linchevski claims is much more natural results.
Looking at supervised machine learning, Linchevski said: “You cannot have results that are not accurate.You need to look at additional layers behind the data, where experienced operators can fit in their knowledge.”
Asked about Boria’s premise that the machine averages out what it learns, rather than identifying best practices, Linchevski described the idea of how a machine can look at production output to identify a golden batch. “If you have a golden batch and you have context, you can take the best period of production and learn from a year of history to understand that something is deviating,” he said. “Machine learning will know [a production run] is not a golden batch.”
Proprietary Industry 4.0
From the people Computer Weekly spoke to, it would appear that industry efforts in machine learning are creating a proprietary Industry 4.0, where companies work on their own unique digital twin simulation software, and gather their own sensor data to feed their machine learning algorithms.
Arguably, this approach is not scalable, and certainly is not the way the packaged software industry evolved to support common business processes. Some industry experts argue that every machine is different, which means each will produce its own set of data to feed its own digital twin.
Speaking ahead of Davos at the opening of HPE’s new IoT innovation lab in Geneva, Phil Davis, president, hybrid IT at Hewlett Packard Enterprise (HPE), said: “I think part of the problem with customers is the amount of data they are collecting. Never throwing away any data is good if you happen to sell storage.” The challenge for customers is knowing what to look for in the data, said Davis.
Tom Bradicich, vice-president and general manager at HPE, said: “People are fearful because there is so much data. We are not sure about the ethical and legal implication of data sharing, and I think this is a strong inhibitor to data sharing.”
While business chiefs and world leaders gather in Davos to hear about the evolution of Industry 4.0, the examples appear to be company-specific, and it is hard to see how Industry 4.0 can scale unless industries adopt a common approach to interoperability and start sharing data.