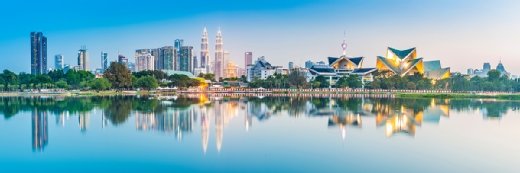
aphotostory - stock.adobe.com
Sea-Lion explained: Southeast Asia’s first large language model
The Sea-Lion large language model was built to cater to the language and cultural diversity of Southeast Asia, which is currently underserved by existing models that mostly originate from the West
In December 2023, Singapore launched a S$70m (US$52m) initiative to build research and engineering capabilities in multimodal large language models (LLMs), including the development of Sea-Lion (Southeast Asian Languages In One Network), the region’s first LLM.
Unlike most other LLMs in the market developed by western tech companies and trained primarily on the corpus of internet content, which is primarily in English, Sea-Lion, built by AI Singapore, is trained on content produced in Southeast Asian languages like Thai, Vietnamese and Bahasa Indonesia.
In turn, Sea-Lion is expected to better understand the context and values related to the diverse cultures and languages of Southeast Asia, such as managing context-switching between languages in multilingual Singapore.
The model, which has been open sourced, is designed to be smaller, more flexible and faster than the commonly used LLMs in the market today. For cost-sensitive and throughput-constrained organisations looking to incorporate artificial intelligence (AI) into their workflows, it can be an inexpensive and more efficient option.
Why is there a need for Sea-Lion?
As a region, Southeast Asia, with a population of over 600 million people, has been underrepresented in the LLMs developed so far. According to the Open LLM leaderboard, the US and China account for the majority of LLMs in the market, with those primarily trained in English making up 60% of the models. Those trained on content in both English and Chinese make up about 27%.
Thus, there’s a need to fill the gap in the market and address the needs of Southeast Asia’s fast-growing digital economy, which is expected to grow from $300bn to almost $1tn by 2030. Unlocking that potential will require LLMs trained in the languages of the region, so that people can not only communicate with machines in their native tongues, but machines will also better understand the context of a user’s prompts and generate higher quality outputs.
How was Sea-Lion built?
Sea-Lion comes in two variants – one with three billion parameters (3B) and another with seven billion parameters (7B). Both variants are built on the MPT (MosaicML Pretrained Transformer) architecture and utilise a vocabulary size of 256K compared to Meta Llama 2’s 32,000. The model employs AI Singapore’s proprietary SEABPETokenizer for tokenisation, specially tailored for Southeast Asian languages, ensuring optimal model performance.
The training data for Sea-Lion is extensive, encompassing one trillion tokens, which equates to 5TB (terabytes) on disk. This vast amount of data has been instrumental in refining the model and enhancing its capabilities.
The data used for pre-training the model was primarily sourced from the internet, specifically the publicly available CommonCrawl dataset. However, much of the data is of low quality, comprising machine translations and keywords optimised for visibility in search engines, according to Leslie Teo, senior director for AI products at AI Singapore. This data had to be cleaned and pre-processed for use in pre-training Sea-Lion.
The proportion of various Southeast Asian languages in the pre-training dataset was also adjusted to reflect the distribution of languages in the region more accurately. This is significant as Southeast Asian content and native languages are underrepresented in pre-training data used for existing LLMs, despite the region being one of the most populous in the world with over 400 million internet users.
To uphold data quality, AI Singapore has a long-term strategy to construct a continuous mining and cleaning pipeline for internet data and will complement its data with those from regional partners. Teo says compared to models like Llama 2, where 0.5% of its data comprises Southeast Asian content, Sea-Lion’s proportion is 13%. “That’s 26 times more, so this is one reason why we have confidence that our model can do better and understand the region better, just by training on higher quality and quantity of data,” he adds.
More importantly, only non-copyrighted data was used to train the model. Teo says although copyrighted material can deliver better performance because of its higher quality, AI Singapore wanted to build a model for people to use without the risk of facing copyright issues. That was also why AI Singapore chose to build the model from scratch as opposed to finetuning an existing model like Llama 2 whose data sources have not been disclosed.
In terms of hardware, Sea-Lion was trained using 256 Nvidia A100 GPUs (graphics processing units) on the Amazon Web Services (AWS) EC2 cloud infrastructure. The training duration for the 3B variant was 14 days, while the more extensive 7B variant required 26 days.
AWS worked closely with AI Singapore to develop Sea-Lion in consultations with the Amazon Science team, as well as its high-performance computing team. It also provided advice on how algorithms could be optimised for efficient training to prevent errors from occurring, meeting customer timelines, and finetuning the model to suit customer needs.
Besides AWS, AI Singapore also worked with Google Research for data and formed partnerships with communities such as SEACrowd to accelerate the creation of a diverse data corpus in native languages. These collaborations aim to develop Sea-Lion’s capabilities and accelerate its adoption by various organisations.
Where is Sea-Lion available?
The model is publicly accessible through platforms such as Hugging Face and GitHub, and will be available in future on AWS SageMaker Jumpstart and Bedrock as well as Google Cloud’s Vertex AI Model Garden. It’s free for research and commercial use to spearhead innovation and use cases across industries, languages and contexts.
The model will focus on commonly used languages in the region, namely Bahasa Indonesia, Malay, Thai and Vietnamese, and will eventually be extended to include other Southeast Asian languages such as Burmese and Lao.
How does Sea-Lion perform for tasks in Southeast Asian languages compared to other LLMs?
Most benchmarks that evaluate the performance of LLMs have largely focused on the English language. In 2023, a team of researchers, mainly from the National University of Singapore, published a paper on an evaluation suite dubbed BHASA that assesses the performance of LLMs in Southeast Asian languages in natural language understanding, generation and reasoning, and translations, among other tasks, and how well they fare in terms of cultural representation and sensitivity.
The benchmark, developed independently of the work that went into training Sea-Lion, found that the Sea-Lion 7B model ranked second behind OpenAI’s GPT-4 in reasoning tasks and translating English into Bahasa Indonesia, but Sea-Lion was better than GPT-4 in understanding sentiment.
Sea-Lion was also trained to provide more concise outputs compared with Llama 2. During a demonstration of how Sea-Lion’s performance compares to other models, where a prompt was posed in Thai on what ASEAN (Association of Southeast Asian Nations) is that required a response in Bahasa Indonesia, Sea-Lion gave an accurate answer in the right language.
Llama 2, on the other hand, did not understand what ASEAN is and gave a lengthier answer in English. Alibaba’s SEA-LLM, which was built on Llama 2 and released weeks after Sea-Lion was launched, understood what ASEAN is but provided false information that Venezuela is part of the regional grouping. Sea-Lion is by no means the best model, and will continue to be improved and finetuned to better cater to the preferences of users in the region.
Who is testing or using Sea-Lion today?
The model will be piloted by companies such as IT service provider NCS and e-commerce company Tokopedia. These companies recognise the value of a region-focused LLM and are working to integrate Sea-Lion to enhance their operations and drive business transformation. For example, the model can be used to power chatbots and translate content such as product descriptions from one Southeast Asian language to another – without using an intermediate language like Chinese or English.
Sea-Lion has also attracted the interest of regional government-linked entities such as Korika, an Indonesian organisation dedicated to advancing AI research and innovation. In Thailand, the Vidyasirimedhi Institute of Science and Technology (Vistec) has also finetuned Sea-Lion with Thai data for its own purposes.
Read more about artificial intelligence in ASEAN
- Google Cloud has teamed up with the Singapore government on a slew of initiatives to drive AI adoption, build an ecosystem of AI startups and expand the pool of AI talent in the city-state.
- Alibaba’s SeaLLMs are built to address the linguistic diversity and nuances in Southeast Asia, enabling businesses to deploy localised chatbots and translation applications.
- Malaysia’s Aerodyne is running its Dronos platform on AWS to expand its footprint globally and support a variety of drone use cases, from agriculture seeding to cellular tower maintenance.
- Healthcare professionals at Singapore’s National University Health System can now summarise patient case notes and predict patient healthcare journeys using a large language model trained by a supercomputer.