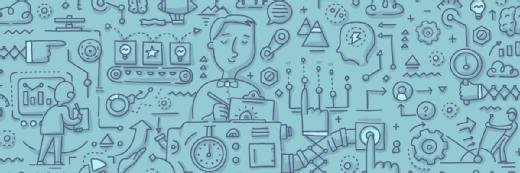
Rassco - FOTOLIA
How AI is helping to drive business process optimisation
Automating an inefficient process simply leads to a faster inefficient process. Artificial intelligence needs to be deployed to ensure business processes are optimal
A key role for any IT leader is to ensure the technology supporting business processes runs as smoothly as possible. Wherever possible, the CIO and a team of enterprise architects and business analysts and consultants also seek to use IT to streamline inefficient or manual processes through business process management (BPM).
A number of technologies have come together to give BPM a new shine. One of these is the use of artificial intelligence (AI) to drive automation. The latest Senior leadership IT investment survey from CCS Insight, which was fielded in July, reports that 80% of firms are now trialling AI or have put it into production. The data shows a significant increase from 2019, when 55% of firms said they were trialling AI.
The Gartner paper Move beyond RPA to deliver hyperautomation identifies a number of areas where artificial intelligence and machine learning (ML) can be used for process automation. The first is to perform continuous learning with data collected in automation processes, which is then used to update models dynamically. The AI makes adjustments to improve the quality of automation.
The second area where AI can be deployed is to re-use learning models that have already been trained on similar datasets. According to Gartner, such models can be repurposed into a new customer’s model, which minimises the time and data required to build and deploy a model.
AI helps businesses through Covid-19
There is a close synergy between business process automation (BPA) and AI, which has become more apparent as businesses try to cope with the Covid-19 coronavirus pandemic. Organisations have had to adapt very quickly and automate those low-hanging fruit manual processes that previously would not have been regarded as a top business priority.
For instance, during the early weeks of the first UK lockdown, the facilities team at insurance firm Generali was tasked with scanning paper documents in the office. The document scanning task is now provided through a managed scanning service.
The result of an automation exercise is a reduction in the volume of manual or inefficient steps required to prepare machine-readable data.
Nicholas McQuire, senior vice-president and head of enterprise and AI research at CCS Insight, believes artificial intelligence is no longer viewed as an experimental, longer-term source of innovation for companies.
“One of the enduring features of the pandemic has been the acceleration we have seen in machine learning in enterprises,” he says.
McQuire believes AI has the potential to deliver quick transformational and business value to help companies automate processes and create new sources of revenue.
Read more about intelligent business process automation
- Business process automation might find new territory to expand into as businesses have become bloated with excess processes since the 2008 crash. But has IT drifted out of business alignment again?
- Artificial intelligence is the next frontier for IT in businesses, helping to increase competitive advantage. A truly successful AI strategy needs careful planning.
In a recent article for Computer Weekly, McQuire described how Australian energy company AGL is using machine learning in a variety of innovative ways to boost automation in its operations.
Many of AGL’s 3.7 million customers use solar power and connected batteries for their household energy. The company has developed a “virtual power plant” product to enable such users to give energy back to the grid. As part of this, AGL has built thousands of machine learning models that help to remotely manage, collect and analyse metadata on energy use from each battery to better understand and forecast capacity across its network.
“Machine learning also automates the process of collecting, feeding and trading the spare capacity as an asset on the national wholesale energy market, generating additional revenue for the company,” says McQuire.
Driving business growth
The environment AGL has built is highly distributed. Each battery provides a rich source of metadata, but the company has also needed to use machine learning due to the stochastic nature of large-scale solar energy data.
AGL used the Microsoft Azure machine learning service for training and inferencing, along with other Kubernetes-based and analytical software to enable a standardised environment for code management, automated machine learning, machine learning operations (MLOps), and real-time performance monitoring and model retraining.
McQuire says AGL’s virtual power plant has not only won several awards in furthering energy sustainability in Australia, but it has also reshaped demand for power in the energy market, essentially rewarding customers for supporting the grid. This promises to improve grid reliability and help customers save on energy bills. AGL claims the underlying architecture has enabled it to train thousands of machine learning models in a 20th of the time normally required.
“What is most fascinating is the level of automation at play, particularly in the potential for trading spare energy on the open market. This aspect would have been impossible on a large scale without machine learning,” he says.
“I recently spoke with David Broeren, AGL’s general manager of integrated energy technology. He highlighted that the provider was adding more datasets, such as snow levels and cloud cover, to improve its forecasting with machine learning. He also hinted at the many new opportunities in expanding the virtual power concept deeper into homes and industries by connecting assets beyond solar batteries to the grid, such as electric vehicles, backup generators and datacentres, for example.”
As McQuire’s AGL example shows, firms operating in the utilities sector have a lot to gain from automation. The nature of the sector means core processes are run on technology that has been built and added to over many years. Often, this no longer offers the most efficient way to run the business. New energy market entrant Goto.energy has grown its customer base by over 200% in just 12 months with the support of Blue Prism’s cloud-based, intelligent digital workforce.
Modernising 40-year-old data flows
The UK’s domestic gas and electricity utility market is data-driven and dominated by six major providers. When it was set up in 2018, Goto.energy mapped out all the business processes required by an energy provider to understand how to start a business in a sector with low margins, says chief financial officer Evan Salway.
As the utilities industry evolved, he says, lots of modules were added to core systems. For instance, businesses did not offer dual-fuel tariffs, so when these were added, they required lots of different data flows which had to be exchanged between industry participants. Some of the back-end systems are 40 years old, based on technology from the early 1980s, such as RPG on AS400 machines.
“We had 200 pages of flowcharts and mapped out where there were duplications,” says Salway. One of the inefficiencies is that there may be hundreds of data flows for a single customer, which often require manual input.
While the average industry time for managing new customer orders is 21 days, Goto.energy is capable of completing the entire process far more quickly. Typically, the bulk of new customer applications received through popular third-party comparison sites is received overnight.
Rather than repeatedly sending variants of the same data flow, Goto.energy has used Blue Prism to create “digital workers”, whereby these data flows are put in a batch process that is submitted to the legacy back-end system and run overnight. In effect, the digital workers verify details against multiple databases using overnight batch processing, which means human workers do not have run the checks manually.
The pre-integrated cognitive capabilities of the Blue Prism digital workers are also being used to eliminate errors and delays in the billing cycle. Tens of thousands of meter readings a month are collected to start the billing process. A large proportion of these are sent in by email and previously had to be keyed into systems manually. The digital workers at Goto.energy read and extract this information automatically. Errors in billing trigger communications back to the customer.
Salway says the company wanted to keep costs low while also offering a high degree of customer service. To differentiate itself from the established competition, he says the company set up a UK-based call centre instead of taking the lower-cost route of running an offshore call centre. “If we are able to use AI and a digital workforce, we can run a UK call centre and get our customer per staff ratio down.”
Optimising processes
As the Goto.energy example shows, robotic process automation can reduce manual steps. AGL is an example of AI being used to power new business processes. AI can also be used to discover new business process optimisations to automate.
Taxi booking app Lyft is one of the examples presented in the Expanding AI’s impact with organisational learning report from Boston Consulting Group in partnership with MIT Sloan Management Review. Lyft uses an algorithm to assess the ride request, where the driver is located and other system dynamics to maximise revenue.
In the report, Lyft’s former vice-president of science, Elizabeth Stone, describes how AI was able to identify a better algorithm. This new algorithm figured out that there is a strong correlation between customers who open the Lyft app and those who end up ordering a taxi via the app.
Similarly, AI can be applied to read legacy application source code line by line to understand what it does. When such insights are combined with AI analysis of the business processes underpinned by the legacy application logic, there are opportunities to modernise the business process, says Munindar Singh, a professor in the department of computer science at North Carolina State University. “You need AI to understand business processes to advise low-level analysis,” he adds.
In effect, any AI-based analysis of the code will have a bearing on the business processes the application powers. This new area of research opens up the possibility of using AI as part of a continuous business process modernisation strategy. In this scenario, algorithms are continually tweaked and the underlying source code modified as the assumptions the AI makes about the business process evolve over time.
Intelligent business process automation could eventually lead to AI systems optimising and redeveloping the code that underpins how businesses operate.