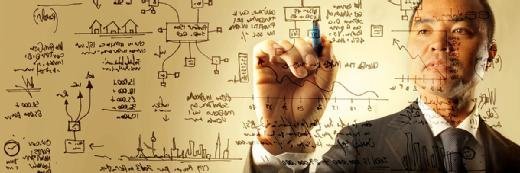
olly - Fotolia
Gain insights into customer behaviour
Predictive analytics can give marketing campaigns a head start, but business stakeholders may need convincing
From an operational perspective, traditional descriptive analytics typically informs universal changes that treat all customers the same, while predictive analytics drives contextualisation and personalised customer interactions.
Companies that adopt predictive analytics require considerable time, planning and cross-functional collaboration to shift from aggregate thinking to customer-specific action.
Deriving value from predictive analytics entails collaborating with key business stakeholders to implement insight-driven programs to win, serve and retain customers.
Unfortunately, according to Forrester’s 2016 State of Customer Analytics survey, 30% of companies rank “getting buy-in from business stakeholders” as one of the key challenges blocking the adoption of analytics.
Contextual marketing engines
Companies that are beginning to adopt predictive analytics tools have not fully thought through how to use these tools to develop contextual marketing engines or personalised customer interactions. Typically, data scientists are not decision makers; effecting operational change is not one of their responsibilities.
This makes it necessary to engage business partners early on. They may not be familiar with predictive analytics or willing to act, so be prepared to educate them and help them translate predictive insights into action.
The process of building a predictive model typically takes from a few weeks to three months, depending on the state and accessibility of the data. This may seem like a long time, but it pales in comparison to the amount of time it takes to adopt and execute personalised marketing successfully.
For this reason, customer insight professionals must design a plan to operationalise predictive insights well before their data scientists start wrangling data. Once they have established a plan for implementation, it will inform each stage of the analytical process to ensure the applicability of the model’s results.
Before setting data scientists loose on the data, Forrester recommends defining the project’s business objective. This may seem simple, but many companies fail to answer some fundamental questions before embarking on predictive analytics, such as: “Why are we doing this? How does it tie into the overall strategy of the organisation?” State the goal of the project in business terms, such as: “The goal of this project is to increase website purchases by existing customers.”
Optimising marketing resources
Read more about data analytics
There is huge demand for data scientists – but rather than build skills in-house, analyst Forrester suggests CIOs can outsource to specialist providers.
Cranfield University, the University of Central Lancashire and the University of Leeds trial one-day course with support from Tableau.
At one US regional bank, the goal for adopting predictive analytics was to develop “a new way of managing and optimising marketing resources around ROI [return on investment]”. This clearly stated goal has led to a 600% ROI by allocating marketing resources more efficiently.
Once the business objectives are set, it is necessary to architect a cross-channel implementation strategy. As predictive analytics gives insights into individual customer behaviour and preferences across channels and touchpoints, it is necessary to have a cross-channel strategy to implement findings. Predictive model results may inform website design, email content and cadence, front-line employee training, and even scripts for call centre staff. The range of applications may seem extensive, but Forrester has found that customer analytics leaders apply insights to about 10 channels – compared with only four for laggards.
The final step is to determine the success metrics. Predetermined success metrics not only help convince leadership, but they also give the project a “true north” to keep it heading in the right direction throughout its life cycle.
If the objective is to predict customer churn, the metric of success should be an increase in customer retention. A company may want to track the retention of high-value customers if it is also analysing customer lifetime value.
Similarly, if the objective is to optimise a marketing campaign, target an increase in response rate as well as healthier attribution metrics. Retailer Costco provides several examples of success metrics; it tracks membership renewals, customer satisfaction, shopping behaviour and sales, and uses these to adjust its sales operations.
One of the most common pitfalls in adopting predictive analytics is a lack of understanding among business partners about how it differs from traditional analytics, how it will benefit them, and what it looks like in action.
To overcome this, it is necessary to explain the analytics mind shift. The move from descriptive to predictive analytics is not merely a time shift from past to future; it is also a mind shift from aggregate analysis to individual treatment, from universal insights to personal insights.
Customer insight professionals need to communicate these differences to business partners, who are likely to be still mired in the world of reporting and dashboarding. Forrester data shows that among global data and analytics technology decision makers, 69% have implemented reporting and 57% have implemented performance analytics, but only 48% have implemented predictive analytics.
Engage business partners early
Forrester also recommends collaborating throughout the analysis. While customer insight managers pros know the value of their predictive analytics initiatives, business partners may not always share that perspective. It is important to engage them early in the process – ideally before beginning any analysis. It may take a couple of months to build a model, but it may take a year to set up the operational infrastructure to deploy that model.
For this reason, companies such as Southwest Airlines and Fujitsu are creating centres of excellence whose mission it is to collaborate with lines of business. This not only ensures buy-in from business owners, but it also opens the lines of communication between them and the modellers – ensuring the development of an actionable predictive model.
Customer insight professionals must come armed with concrete suggestions for taking action based on predictive analytics outcomes, especially when the language of predictive analytics is foreign to many business partners not formally trained in analytics. This requires a solid understanding of how to translate analytical outputs into action, often in an automated fashion.
Predictive analytics allows marketers to send the right messages to the right customers at the right time. Most customer insight leaders will be familiar with the story of US retailer Target knowing a teenage girl was pregnant before her father did – but many firms have yet to use customer segmentation to optimise marketing campaigns. Businesses that do so are seeing quick benefits.
For example, adventure and outdoor brand The Clymb increased its revenue by 175% per email sent and increased its total email revenue by 71% using predictive personalised email campaigns.
This is an excerpt from the Forrester report, Close the insights-to-action gap with a clear implementation plan, by senior analyst Brandon Purcell.