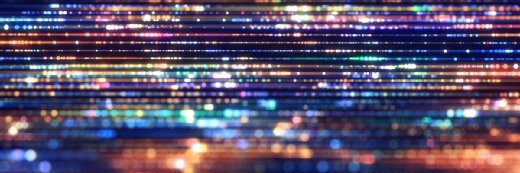
Bokehstore - stock.adobe.com
Data integration dogged by complexity and vital to business
Integrating data is one of the thorniest challenges in business intelligence and analytics – achieving it is technically and organisationally complex, while it is gaining in importance all the time
Integrating data is arguably one of the most pressing challenges in business intelligence and analytics at present.
Organisations are dealing with seemingly endless increases in the volume of data they handle. Analytics teams are under pressure to deliver information and insights to the business more quickly while also dealing with a wider range of data sources.
Often, today’s data sources are disconnected, use different data classifications, are delivered at different speeds, and differ widely in data quality. Yet organisations’ data scientists and analysts need to combine these sources in a way that allows business users to form a consistent, accurate data picture that supports better decision-making.
The volume of data, and its velocity, means manual integration is all but impossible, save for the smallest projects and prototypes. Instead, firms are looking for processes that clean and integrate datasets before passing them on to analysis, business intelligence (BI), or even machine learning (ML) tools.
But even then, organisations mush deal with different teams using datasets running across fragmented but often overlapping integration tools.
And with different approaches to integration, including replication, synchronisation and data virtualisation, the market is only now starting to move towards technologies that can handle all of an enterprise’s data integration needs in one place.
“The most important reason for data integration, and one of the main reasons people struggle with their data analysis initiatives, is because they don’t integrate their data,” warns Ehtisham Zaidi, an analyst covering data management at Gartner.
Difficult though it is, that integration is vital if organisations are going to recoup their investment in collecting, storing and managing their data in the first place.
Data integration and business goals
As Gartner’s Zaidi points out, data sharing, inside and outside the enterprise, is increasingly important. As is the need to gather and analyse operational and transactional data, and to support emerging tools such as machine learning and artificial intelligence.
Firms have taken to harvesting ever larger volumes of data from their transactional systems, software-as-a-service (SaaS) applications, e-commerce, social media, sensors and the internet of things (IoT). The global volume of data created was 2ZB (zettabytes) in 2010, but 10 years later it was 64ZB. By 2025 it could be 181ZB, according to analysts at Statista.
Much of that data is mostly static, such as archival information and backups. As Statista points out, the rapid growth of data volumes during the Covid-19 pandemic was driven, in part, by the need for employees to copy files so they could work from home.
But that still leaves a vast amount of “live” data that organisations want to process in their BI, predictive analytics and other insight tools, alongside data retained for regulatory purposes.
Businesses across all sectors talk about being “data driven”, whether they make jet engines or the humble pizza. Rolls-Royce uses a system based primarily on Microsoft Azure cloud services to monitor jet engine performance. Domino’s Pizza uses software from Talend to integrate some 85,000 data sources.
“Data integration is the ability to capture and transform data from multiple sources, and combine it to gain insights,” says Michele Goetz, an analyst covering data management and business intelligence at Forrester.
Combining data sources allows firms to view their operations from different angles, whether customer engagement or business processes, and to do so faster than ever.
“Being able to capture data from multiple points is extremely important,” she says. “By not capturing from multiple sources, by not integrating and rationalising that data together, there are a lot of blind spots in your business. That impacts your decision-making ability.”
Creating this full picture means having accurate, clean and compatible data. But if organisations want to exploit the insights from their data, they need to integrate it in a timely manner. Goetz ranks this “freshness” along with accuracy – data needs to be both relevant and timely. And this means automating data integration.
The practice of data integration
The conventional, and still common, way to integrate data is ETL: extract, transform and load. Here, the data is brought in from the disparate systems, transformed – cleaned and converted if needed to a common data taxonomy – and then loaded into the next system along. This could be a database, data warehouse or BI application.
But this approach struggles with the growing range of data sources and the need for ever quicker responses.
“The notion that, ‘as long as I can gather the data in a data warehouse, it will satisfy 80-90% of needs’ is not viable anymore, if you are trying to keep up with demands that are changing second by second,” says Goetz.
Data risks losing its freshness and relevance. Instead of a working tool for the business, conventional approaches risk creating a “data museum” – useful, perhaps, for looking at past performance, but not for real-time or predictive analytics.
ETL still has a role to play in workflows that allow batch processing, or can run overnight. According to Gartner’s Zaidi, however, ETL is being complemented by what he calls “more modern ways of data integration”. Those are data replication, data synchronisation and data virtualisation.
These approaches allow analysts to process data without having to move it, speeding up the process, reducing reworking, and allowing more flexibility. In addition, organisations might need to deal with streamed data, and more modern tools can integrate event data or logs.
Whichever approach is taken, the objective is to create a dataset once that can be used many times, without reformatting or loading into a new system. In turn, this has created its own problem – a proliferation of data integration tools.
But there are signs that the market is starting to consolidate.
Data integration: maturity and consolidation
At one level, data integration is a paradox. It brings together disparate data sources and IT systems, but different approaches to integration have led to a proliferation of disparate, and often incompatible, tools.
Gartner, for example, states that “in large enterprises, different teams [use] different tools, with little consistency, lots of overlap and redundancy, and no common management or leverage of metadata”.
Mike Ferguson, managing director of Intelligent Business Strategies, describes the problem. “If you create something in one tool, I can’t pick it up and then run it in another tool,” he says. “And if you’re a global organisation, because business departments are quite autonomous, they go off and buy their own toolsets. So big organisations end up with a range of different tools.”
Suppliers are moving to address this through market consolidation. Those best known for ETL and data warehouse technology are broadening their product sets to include replication, synchronisation and virtualisation.
Open source remains an option for firms with the time and know-how to build their own integration platforms. But Gartner’s Zaidi says this is rarely necessary. “The market for end user tools has matured,” he says. “There are mature vendors providing battle-tested tools. They can all handle these new ways of integration.”
Companies in the market include Confluent, Informatica, Talend, Tibco and IT industry stalwarts IBM and Oracle, to name just a few.
Firms can invest in off-the-shelf integration tools and put data analysis into the hands of business users and data scientists rather than IT teams or developers. But even with an improving and better-integrated toolset, challenges remain.
Data integration: the remaining challenges
Software tools alone will not address all the challenges posed by data integration.
Firms looking to maximise the value of their business data must still address data quality and integrity, provide consistent and timely data flows into BI and analytics applications, and ensure that business leaders act on the insights from those applications. And they need to do this with skills that remain in short supply.
“Data quality is still a massive problem,” says Mike Ferguson. “And as more and more data sources become available, it becomes almost a never-ending one.”
There are governance issues too, because data resides in more places, in more formats. “It is far more difficult to govern data than it ever used to be because we now have so many sources,” he says.
Intelligent integration helps firms extract value from their data and, as Ferguson notes, automation helps address the skills deficit or, at least, allows users in the business who are not specialist data scientists to start pulling together data sources for analysis.
“It’s all about speeding up development, by getting more people able to [manage data] and lowering the skills bar by doing more in the way of automation,” he says.
Tools should also give better visibility of data assets. But they will not, on their own, address data quality or governance issues. Master data management policies, strong data science capabilities and, potentially, a chief data officer (CDO) with a seat on the board are all needed as well.
“In the past, you could put all your data in one place,” says Forrester’s Goetz. “We had the systems that were suited to recognising that there is a limited amount of information you need, and that is what you are going to harness and use.
“Today, because of the way we drive our business, through digital ecosystems…you have to be much more flexible and adapt to knowing what data you need, where that data resides and how you best integrate it, at the right time, at the right freshness, so that information is relevant.”
Read more about data integration
- What is data integration?
- Data integration platforms take users beyond ETL software.
- Data integration vs. ETL in the age of big data.