TIBCO CTO Petracek: Getting the fuel mix right to feed the AI engine
Not all software is equal.
Some software is just software i.e. it’s application (apps), components or perhaps even mircoservices.
Some software is higher-level software i.e. it’s platform-level (think IDE and complete language framework) software designed to provide functionality but also conduits to other tributary elements of code and more.
So software is software that beats it out like a workhorse i.e. these are the software ‘engines’ that we talk about when we think about the analytics-centric processing intelligence that Artificial Intelligence (AI) engines have to pull off in order to deliver the insight that we demand of them.
Global CTO of software integration and analytics company TIBCO Nelson Petracek explains that his company understands the rainbow nation of different data structures and different deployment models that exists out there.
Specifically when it comes to juggling the (often GPU-based) processing power needed for AI, Petracek has been quoted saying that when it comes to AI model development and deployment, organizations are concerned with a number of issues, many of which will centralise around the need to collect, prepare, catalog, store and access all of the data needed for the AI models to be run.
So what does Petracek see as some of the main challenges that AI engines face when organisations are trying to make sure that they are fed with enough fuel to do their job properly… is there a recipe for success?
Feeding the AI engine
TIBCO’s Petracek notes that the speed and accuracy over which an analytics pipeline can be executed (where he defines the “pipeline” here as a set of simplified high-level stages, including data discovery, data retrieval, data science/ML, model deployment/ops, visual analytics & interaction) is a key concern when organisations are looking to allocate appropriate resources to AI.
“[Among other must-haves for AI operationalisation are] model development and associated concerns such as model performance, model explainability and model operationalisation. Companies need to think about the cost of the steps above (infrastructure, people, time, opportunity cost)… and they need to consider the speed and cost of retraining cycles and identifying when this needs to occur (or when rebaselining needs to occur in unsupervised learning, for example). Further, they need to consider prioritisation of model development, training, and operationalisation across business needs, based on considerations such as model value and business criticality,” noted TIBCO’s Petracek, in a briefing analysis with the Computer Weekly Developer Network.
Model visibility
He also notes the need to think about visibility into model performance for the purposes of measuring business value vs. the cost of developing and maintaining the model.
“I obviously have lots of ‘speed’, ‘cost’, ‘efficiency’ and ‘time’ components to my points above. This is where techniques that allow for the more efficient use of hardware resources come into play. Yes, it is about optimising the cost and usage of the infrastructure (cloud or on-premises), but it also comes down to the ‘why’ of doing so… and to the level of transparency needed by the technology itself (e.g. what extra effort is required on the part of the users to take advantage of these techniques). This is why determining the value of a model is so important,” said Petracek.
He reminds us that just because you ‘can’ train and deploy a model faster doesn’t mean that you ‘should’.
Of course, being able to determine the value of a model faster has obvious benefits, as does recognising when a model is not performing as expected and subsequently making adjustments (rerunning the cycle).
In closing, Petracek thinks that in today’s world (even if one sets aside the current global COVID-19 concern), the context of data analytics and wider elements of AI is continuously changing and organisations that react faster and with more accuracy to these changes will have a greater chance of success.
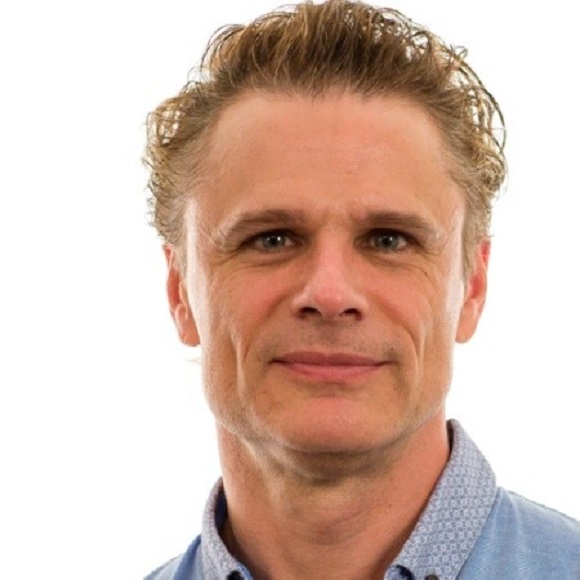
TIBCO CTO Nelson Petracek: This year’s (data) model is shaping up.