Data engineering - Alteryx: Investing for scale with an eye on value
This is a guest post for the Computer Weekly Developer Network written by Alan Jacobson in his role as chief data & analytics officer at Alteryx.
Bata engineering is a crucial aspect of a data-driven organisation, but it doesn’t create any value of its own… rather, it underpins the value-driving operations, creating the precarious position of discerning the delicate balance between justifiable investment in data engineering and tangible business value creation.
Jacobson takes this reality forward and explains how organisations can harness data engineering – at scale – with an inscrutable eye on value at the same time… he wries in full as follows.
Value parameters
Data only delivers value when it is used – perhaps by a domain expert solving a problem or a data scientist creating analytics for the business. Misjudging this equilibrium and investing too heavily in data engineering before delivering significant value can spell an early demise.
Data engineering provides the infrastructure necessary to harness vast amounts of data, fuelling transformative pillars such as business analytics, machine learning and decision-making processes. In many cases, business problems can be solved without large-scale data engineering efforts, however, it will take more time and effort. Data engineering, therefore, remains a somewhat misunderstood cost centre with a hazy return on investment.
Data engineers create, manage and optimise data lakes and warehouses where data is transformed, cleaned and structured appropriately to feed into revenue-producing or cost-saving processes. Costly infrastructures to overcome time management constraints and operational complexity must be justified without being detrimental to downstream investments in analytical applications and decision-making tools.
Achieving a symbiotic relationship between data engineering investment and business use-case execution through parallel investment is key. Taking this approach and avoiding early overinvestment in data engineering ensures applications are informed and guided by the value delivery process.
Avoiding the single-source fallacy
The ‘single source of truth’ is a common trope in data engineering, but it is largely a myth. Some enquiries only need a single answer, but most questions are diverse and varied depending on why the question is being asked. For example, a user asking “how many blue widgets were sold last week” might be asking about the last business week or the last seven days and ‘sold’ could mean an order placed or cash received.
Rigidly enforcing a sole data source and definition for an organisation could spell disaster. Even a well-engineered lake will not encompass all the variations of every question that comes in day-to-day. A great data ecosystem will typically host 30% – 70% of data required for an enquiry, leaving a large amount for analysts to source from elsewhere. Forcing exclusive dependence on sources built by a data engineering team will drive users to find other solutions, undermine the collaboration of teams and inadvertently pave the road to shadow IT. The repercussions of this are slow responses to management questions or an absence of data from answers that deserve to be data-driven.
As analytic needs are fluid, data engineers must work closely with business users. This ensures that business use cases can securely draw on diverse data. Over time, the ratio of centrally managed data versus other sources is likely to increase to create a more integrated environment.
So, how can businesses ensure all-important data engineering investment is as efficient as possible? The world is moving towards a more open and autonomous way of operating which will help cut costs while boosting efficiency. By shifting in that direction and implementing best practices and technology into operations, effective data engineering at scale is in much closer reach.
Embrace open & best-of-breed
Flexibility is crucial. Data engineering environments must be able to integrate and operate seamlessly within modern toolchains and workflows. Open, extensible environments allow for the use of best-of-breed tools, enabling rapid problem-solving and innovation while avoiding vendor lock-in.
Using machine learning and AI to reduce manual data engineering tasks and eliminate human error allows data engineers to focus on strategic initiatives rather than manual grunt work. Machine learning tools offer predictive transformation suggestions and adaptive data quality assessments to streamline data pipeline operations.
Democratise data across teams
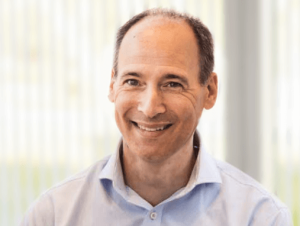
Alteryx’s Jacobson: Forcing singular dependence on sources built by a data engineering team will drive users to find other solutions, undermine collaboration & and inadvertently pave the road to shadow IT.
Data democratisation boosts collaboration and speeds up processing. By breaking down data silos and enabling access, actionable insights can be generated faster by teams across the business. Democratisation should involve both technical and non-technical team members to foster a culture of shared responsibility when it comes to data handling and data championing. Selecting tools that can be used beyond the data engineering team is essential here as all knowledge workers are data workers in today’s modern company.
As data volumes continue to multiply, scalability and governance at scale must be core considerations. They cannot be implemented as afterthoughts. Finding solutions that can be deployed with strong data security, encryption and compliance is critical for robust, future-proof data infrastructures.
Balancing the paradox
Data engineering is a critical function in efficiently and safely using data, but it is also a cost centre.
Data engineering won’t pay the bills so must be coupled with projects and teams that are delivering value with the data to outpace engineering spending.
This will ensure that costs are balanced and downstream business uses are delivered so data can be used to truly transform.